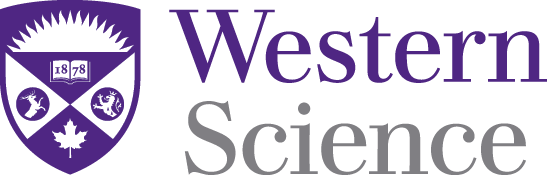
Statistical and Actuarial Sciences Publications
Document Type
Article
Publication Date
1-6-2021
Journal
The Journal of Credit Risk
Volume
16
Issue
4
First Page
119
Last Page
156
URL with Digital Object Identifier
https://doi.org/10.21314/JCR.2020.272
Abstract
The main aim of this study is to analyse the joint effects of customer segmentation, borrowers' characteristics and modelling techniques on the classification accuracy of a scoring model for agribusinesses. To this end, we used data provided by a Chilean company on 161,163 loans from January 2007 to December 2013. We considered random forest, neural network and logistic regression models as analytical methods. Regarding the borrowers' profiles, we examined the effects of socio-demographic, repayment-behaviour, agribusiness-specific and credit-related variables. We also segmented the customers as individuals, SMEs and large holdings. As the segments show different risk behaviours, we obtained a better performance when we estimated a scoring model for each segment instead of using a segmentation variable. In terms of the value of each set of variables, behavioural variables increased the predictive capability of the model by double the amount achieved by including agribusiness-related variables. The random forest is the model with the best classification accuracy.
Creative Commons License
This work is licensed under a Creative Commons Attribution-Noncommercial-No Derivative Works 4.0 License.
Included in
Agribusiness Commons, Business Analytics Commons, Entrepreneurial and Small Business Operations Commons, Operational Research Commons, Risk Analysis Commons