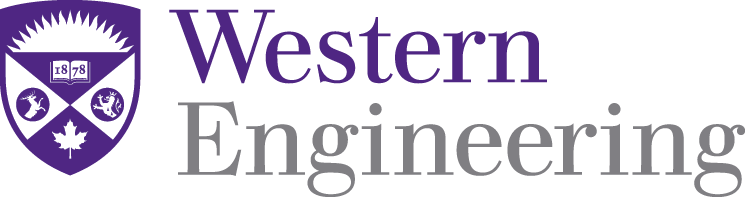
Mechanical and Materials Engineering Publications
Document Type
Article
Publication Date
1-1-2021
Volume
29
Journal
IEEE Transactions on Neural Systems and Rehabilitation Engineering
First Page
1413
URL with Digital Object Identifier
10.1109/TNSRE.2021.3097007
Last Page
1423
Abstract
Wearable tremor suppression devices (WTSD) have been considered as a viable solution to manage parkinsonian tremor. WTSDs showed their ability to improve the quality of life of individuals suffering from parkinsonian tremor, by helping them to perform activities of daily living (ADL). Since parkinsonian tremor has been shown to be nonstationary, nonlinear, and stochastic in nature, the performance of the tremor models used by WTSDs is affected by their inability to adapt to the nonlinear behaviour of tremor. Another drawback that the models have is their limitation to estimate or predict one step ahead, which introduces delay when used in real time with WTSDs, which compromises performance. To address these issues, this work proposes a deep neural network model that learns the correlations and nonlinearities of tremor and voluntary motion, and is capable of multi-step prediction with minimal delay. A generalized model that is task and user-independent is presented. The model achieved an average estimation percentage accuracy of 99.2%. The average future voluntary motion prediction percentage accuracy with 10, 20, 50, and 100 steps ahead was 97.0%, 94.0%, 91.6%, and 89.9%, respectively, with prediction time as low as 1.5 ms for 100 steps ahead. The proposed model also achieved an average of 93.8% ± 1.5% in tremor reduction when it was tested in an experimental setup in real time. The tremor reduction showed an improvement of 25% over the Weighted Fourier Linear Combiner (WFLC), an estimator commonly used with WTSDs.
Creative Commons License
This work is licensed under a Creative Commons Attribution-Noncommercial-No Derivative Works 4.0 License.