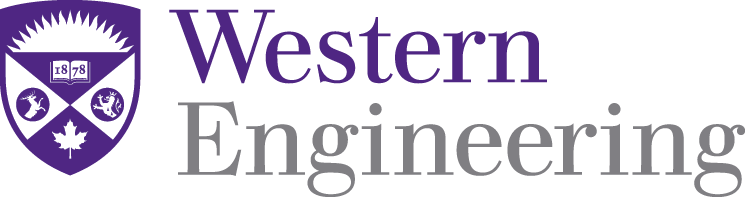
Electrical and Computer Engineering Publications
Document Type
Article
Publication Date
2024
Journal
IEEE Sensors
URL with Digital Object Identifier
10.1109/JSEN.2024.3451291
Abstract
When humans repeat the same motion, the tendons, muscles, and nerves can be damaged, causing Repetitive Stress Injuries (RSI). If the repetitive motions that lead to RSI are recognized early, actions can be taken to prevent these injuries. As Human Activity Recognition (HAR) aims to identify activities employing wearable or environment sensors, HAR is the first step toward identifying repetitive motions. Deep learning models, such as Convolutional Neural Networks (CNNs), have seen great success in recognizing activities for participants whose data are used in the model training; however, their accuracy drops for new participants as people move in different ways. Moreover, most studies focus on lower body movement, while upper body movements are the main cause of RSI. On the other hand, in recent years, transformers have been dominating natural language processing, and have the potential to improve modelling in other domains involving sequential data such as HAR. Consequently, this paper combines a Transformer and CNN (Trans-CNN) for the recognition of upper and lower body movements. Transfer learning was employed to personalize the generic model for the target participant. The experiments demonstrate that the generic Trans-CNN outperforms the standalone transformer and CNN. The accuracy of the generic Trans-CNN for both upper and lower body movements improved from 69.6% to 92.4% when personalization was introduced. All models, irrespective of the algorithm, have more difficulty recognizing upper body than lower body movements. Nevertheless, the proposed personalized approach for the detection of upper and lower body movements represents significant progress toward RSI prevention.
Included in
Artificial Intelligence and Robotics Commons, Computer Engineering Commons, Data Science Commons, Electrical and Computer Engineering Commons