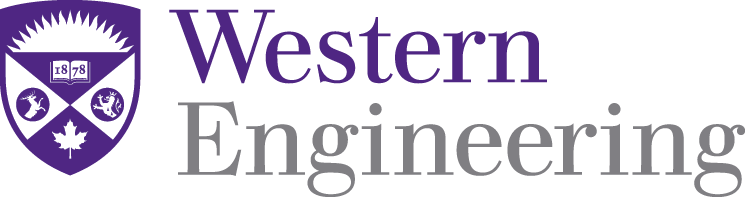
Electrical and Computer Engineering Publications
Document Type
Article
Publication Date
1-1-2022
Volume
30
Journal
IEEE Transactions on Neural Systems and Rehabilitation Engineering
First Page
2856
URL with Digital Object Identifier
10.1109/TNSRE.2022.3211450
Last Page
2865
Abstract
The side effects and complications of traditional treatments for treating pathological tremor have led to a growing research interest in wearable tremor suppression devices (WTSDs) as an alternative approach. Similar to how the human brain coordinates the function of the human system, a tremor estimator determines how a WTSD functions. Although many tremor estimation algorithms have been developed and validated, whether they can be implemented on a cost-effective embedded system has not been studied; furthermore, their effectiveness on tremor signals with multiple harmonics has not been investigated. Therefore, in this study, four tremor estimators were implemented, evaluated, and compared: Weighted-frequency Fourier Linear Combiner (WFLC), WFLC-based Kalman Filter (WFLC-KF), Band-limited Multiple FLC, and enhanced High-order WFLC-KF (eHWFLC-KF). This study aimed to evaluate the performance of each algorithm on a bench-top tremor suppression system with 18 recorded tremor motion datasets; and compare the performance of each estimator. The experimental evaluation showed that the eHWFLC-KF-based WTSD achieved the best performance when suppressing tremor with an average of 89.3% reduction in tremor power, and an average error when tracking voluntary motion of 6.6°/s. Statistical analysis indicated that the eHWFLC-KF-based WTSD is able to reduce the power of tremor better than the WFLC and WFLC-KF, and the BMFLC-based WTSD is better than the WFLC. The performance when tracking voluntary motion is similar among all systems. This study has proven the feasibility of implementing various tremor estimators in a cost-effective embedded system, and provided a real-time performance assessment of four tremor estimators.