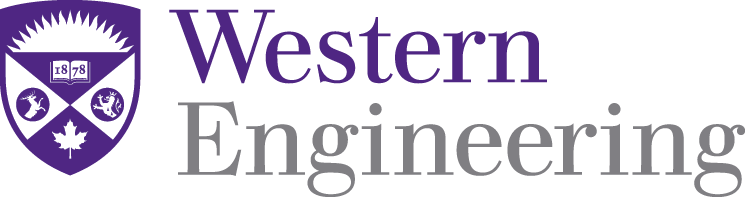
Electrical and Computer Engineering Publications
Document Type
Article
Publication Date
10-16-2023
Journal
49th Annual Conference of the IEEE Industrial Electronics Society
First Page
1
URL with Digital Object Identifier
10.1109/IECON51785.2023.10311616
Last Page
7
Abstract
This paper explores the role of continual learning strategies when neural networks are confronted with learning tasks sequentially. We analyze the stability-plasticity dilemma with three factors in mind: the type of network architecture used, the continual learning scenario defined and the continual learning strategy implemented. Our results show that complementary learning systems and neural volume significantly contribute towards memory retrieval and consolidation in neural networks. Finally, we demonstrate how regularization strategies such as elastic weight consolidation are more well-suited for larger neural networks whereas rehearsal strategies such as gradient episodic memory are better suited for smaller neural networks.
Creative Commons License
This work is licensed under a Creative Commons Attribution-Share Alike 4.0 License.
Citation of this paper:
Christopher Tam and Luiz Fernando Capretz, Investigating Continual Learning Strategies in Neural Networks, IECON - 49th Annual Conference of the Industrial Electronics Society, Singapore, pp. 1-7, October 2023. DOI: https://doi.org/10.1109/IECON51785.2023.10311616.
Notes
IECON - 49th Annual Conference of the Industrial Electronics Society, Singapore, pp. 1-7, October 2023. DOI: https://doi.org/10.1109/IECON51785.2023.10311616