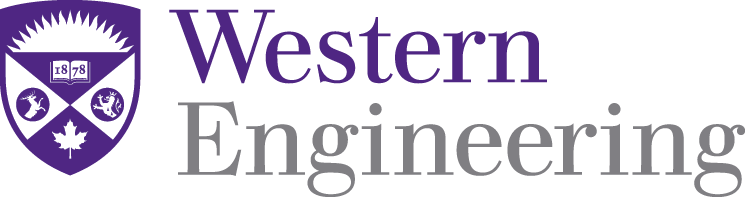
Electrical and Computer Engineering Publications
Document Type
Article
Publication Date
2021
Volume
14
Issue
12
Journal
Energies
URL with Digital Object Identifier
https://doi.org/10.3390/en14123623
Abstract
High-impedance faults (HIF) are difficult to detect because of their low current amplitude and highly diverse characteristics. In recent years, machine learning (ML) has been gaining popularity in HIF detection because ML techniques learn patterns from data and successfully detect HIFs. However, as these methods are based on supervised learning, they fail to reliably detect any scenario, fault or non-fault, not present in the training data. Consequently, this paper takes advantage of unsupervised learning and proposes a convolutional autoencoder framework for HIF detection (CAE-HIFD). Contrary to the conventional autoencoders that learn from normal behavior, the convolutional autoencoder (CAE) in CAE-HIFD learns only from the HIF signals eliminating the need for presence of diverse non-HIF scenarios in the CAE training. CAE distinguishes HIFs from non-HIF operating conditions by employing cross-correlation. To discriminate HIFs from transient disturbances such as capacitor or load switching, CAE-HIFD uses kurtosis, a statistical measure of the probability distribution shape. The performance evaluation studies conducted using the IEEE 13-node test feeder indicate that the CAE-HIFD reliably detects HIFs, outperforms the state-of-the-art HIF detection techniques, and is robust against noise.
Creative Commons License
This work is licensed under a Creative Commons Attribution 4.0 License.
Citation of this paper:
Rai, K.; Hojatpanah, F.; Badrkhani Ajaei, F.; Grolinger, K. Deep Learning for High-Impedance Fault Detection: Convolutional Autoencoders. Energies 2021, 14, 3623. https://doi.org/10.3390/en14123623
Notes
https://doi.org/10.3390/en14123623