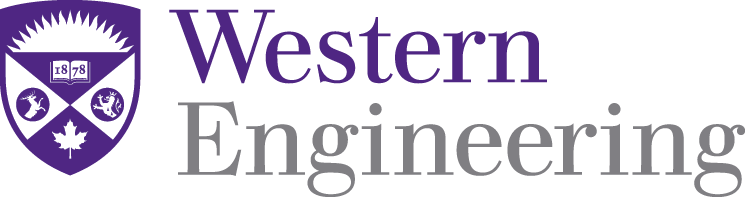
Electrical and Computer Engineering Publications
Energy Disaggregation Using Multilabel Binarization and Gaussian Naive Bayes Classifier
Document Type
Conference Proceeding
Publication Date
10-2020
First Page
0093
URL with Digital Object Identifier
10.1109/UEMCON51285.2020.9298157
Last Page
0100
Abstract
Nearly everywhere across the globe, energy demand is increasing, as new residential buildings emerge and population growth continues. It is important to meet the energy demand while still generating less energy to make up for what is needed and energy disaggregation can assist in this process. In this work, we propose an energy disaggregation method that would allow consumers to be informed on the state (on or off) of their appliances at any point in time. This would enable them to adjust their appliance usage thereby consuming less energy. Our disaggregation approach can disaggregate any combination of appliances among a set of appliances. This is done by creating and fitting n-models for the n-combinations possible in a strategic manner. Prior to the models, Multilabel Binarization has been used to deduce the labels of the data, then the models are developed and fitted using Gaussian Naive Bayesian Classifier. Thereafter, with Jaccard Coefficient per appliance and accuracy, recall, precision and f1-score were used for overall score. We evaluate these models with the original and noisy synthesized test data, resulting in an average overall F1-score of 91% for the original and 87.8% for the synthesized test data.
Citation of this paper:
P. Adjei, N. S. Sethi, C. P. E. de Souza and M. A. M. Capretz, "Energy Disaggregation using Multilabel Binarization and Gaussian Naive Bayes Classifier," 2020 11th IEEE Annual Ubiquitous Computing, Electronics & Mobile Communication Conference (UEMCON), 2020, pp. 0093-0100, doi: 10.1109/UEMCON51285.2020.9298157.
Notes
https://ieeexplore.ieee.org/document/9298157