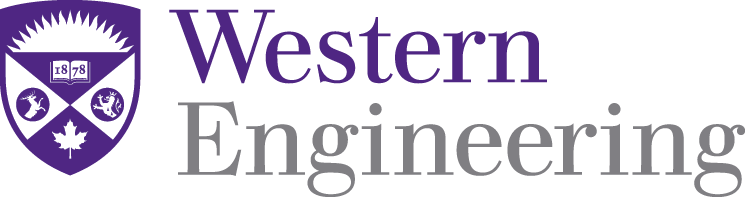
Electrical and Computer Engineering Publications
A Systematic Review of Convolutional Neural Network-Based Structural Condition Assessment Techniques
Document Type
Article
Publication Date
2021
Volume
226
Journal
Engineering Structures
URL with Digital Object Identifier
10.1016/j.engstruct.2020.111347
Abstract
With recent advances in non-contact sensing technology such as cameras, unmanned aerial and ground vehicles, the structural health monitoring (SHM) community has witnessed a prominent growth in deep learning-based condition assessment techniques of structural systems. These deep learning methods rely primarily on convolutional neural networks (CNNs). The CNN networks are trained using a large number of datasets for various types of damage and anomaly detection and post-disaster reconnaissance. The trained networks are then utilized to analyze newer data to detect the type and severity of the damage, enhancing the capabilities of non-contact sensors in developing autonomous SHM systems. In recent years, a broad range of CNN architectures has been developed by researchers to accommodate the extent of lighting and weather conditions, the quality of images, the amount of background and foreground noise, and multiclass damage in the structures. This paper presents a detailed literature review of existing CNN-based techniques in the context of infrastructure monitoring and maintenance. The review is categorized into multiple classes depending on the specific application and development of CNNs applied to data obtained from a wide range of structures. The challenges and limitations of the existing literature are discussed in detail at the end, followed by a brief conclusion on potential future research directions of CNN in structural condition assessment.
Included in
Civil and Environmental Engineering Commons, Computer Engineering Commons, Electrical and Computer Engineering Commons