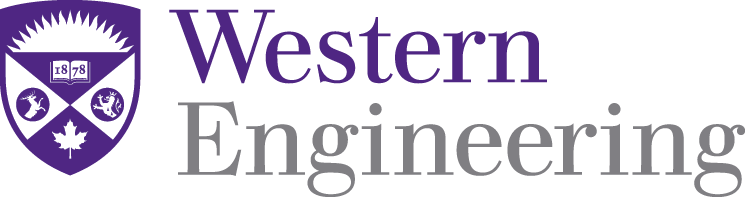
Electrical and Computer Engineering Publications
Document Type
Article
Publication Date
2017
Volume
144
Journal
Energy and Buildings
First Page
191
URL with Digital Object Identifier
http://doi.org/10.1016/j.enbuild.2017.02.058
Last Page
206
Abstract
During building operation, a significant amount of energy is wasted due to equipment and human-related faults. To reduce waste, today's smart buildings monitor energy usage with the aim of identifying abnormal consumption behaviour and notifying the building manager to implement appropriate energy-saving procedures. To this end, this research proposes a new pattern-based anomaly classifier, the collective contextual anomaly detection using sliding window (CCAD-SW) framework. The CCAD-SW framework identifies anomalous consumption patterns using overlapping sliding windows. To enhance the anomaly detection capacity of the CCAD-SW, this research also proposes the ensemble anomaly detection (EAD) framework. The EAD is a generic framework that combines several anomaly detection classifiers using majority voting. To ensure diversity of anomaly classifiers, the EAD is implemented by combining pattern-based (e.g., CCAD-SW) and prediction-based anomaly classifiers. The research was evaluated using real-world data provided by Powersmiths, located in Brampton, Ontario, Canada. Results show that the EAD framework improved the sensitivity of the CCAD-SW by 3.6% and reduced false alarm rate by 2.7%.
Included in
Artificial Intelligence and Robotics Commons, Computer Engineering Commons, Electrical and Computer Engineering Commons, Software Engineering Commons