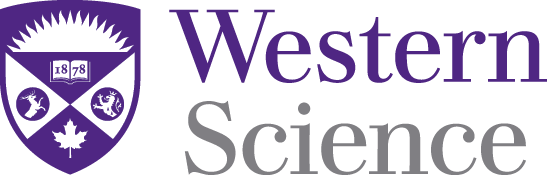
Computer Science Publications
Title
Machine Learning towards Enabling Spectrum-as-a-Service Dynamic Sharing
Document Type
Conference Proceeding
Publication Date
8-30-2020
Volume
2020-August
Journal
Canadian Conference on Electrical and Computer Engineering
URL with Digital Object Identifier
10.1109/CCECE47787.2020.9255817
Abstract
© 2020 IEEE. The growth in wireless broadband users, devices, and novel applications has led to a significant increase in the demand for new radio frequency spectrum. This is expected to grow even further given the projection that the global traffic per year will reach 4.8 zettabytes by 2022. Moreover, it is projected that the number of Internet users will reach 4.8 billion and the number of connected devices will be close 28.5 billion devices. However, due to the spectrum being mostly allocated and divided, providing more spectrum to expand existing services or offer new ones has become more challenging. To address this, spectrum sharing has been proposed as a potential solution to improve spectrum utilization efficiency. Adopting effective and efficient spectrum sharing mechanisms is in itself a challenging task given the multitude of levels and techniques that can be integrated to enable it. To that end, this paper provides an overview of the different spectrum sharing levels and techniques that have been proposed in the literature. Moreover, it discusses the potential of adopting dynamic sharing mechanisms by offering Spectrum-as-a-Service architecture. Furthermore, it describes the potential role of machine learning models in facilitating the automated and efficient dynamic sharing of the spectrum and offering Spectrum-as-a-Service.