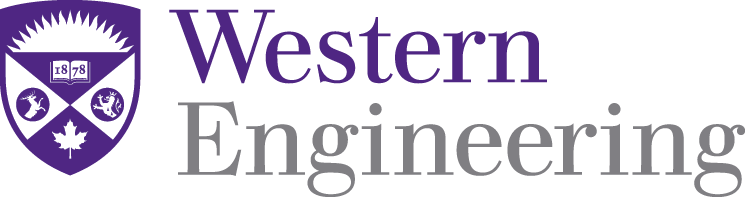
Civil and Environmental Engineering Publications
Document Type
Article
Publication Date
2021
Creative Commons License
This work is licensed under a Creative Commons Attribution-Noncommercial-No Derivative Works 4.0 License.
Abstract
In this paper, a novel method is proposed based on a windowed-one-dimensional convolutional neural network for multiclass damage detection using acceleration responses. The data is pre-processed and augmented by extracting samples of windows of the original acceleration time series. 1D CNN is developed to classify the signals in multiple classes. The damage is detected if the predicted classification is one of the indicated damage levels. The damage is quantified using the predicted class probabilities. Various signals from the accelerometers are provided as input to the 1D CNN model, and the resulting class probabilities are used to identify the location of the damage. The proposed method is validated using Z24 bridge benchmark data for multiclass classification for two damage scenarios. The results show that the proposed 1D CNN method performs with superior accuracy for severe damage cases and works well with different types of damage types.