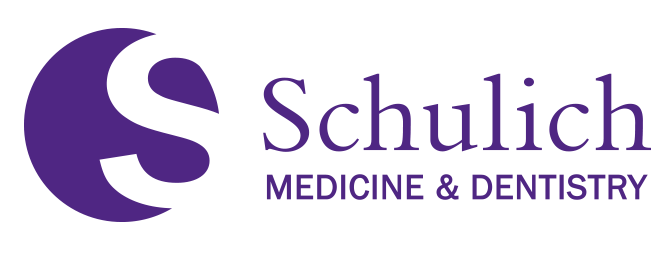
Biochemistry Publications
Document Type
Article
Publication Date
11-15-2020
Journal
BioRxiv
URL with Digital Object Identifier
10.1101/2020.11.13.381798
Creative Commons License
This work is licensed under a Creative Commons Attribution-Noncommercial 4.0 License
Citation of this paper:
Pathway-extended gene expression signatures integrate novel biomarkers that improve predictions of patient responses to kinase inhibitors Ashis J. Bagchee-Clark, Eliseos J. Mucaki, Tyson Whitehead, Peter K. Rogan bioRxiv 2020.11.13.381798; doi: https://doi.org/10.1101/2020.11.13.381798
Included in
Biochemistry Commons, Bioinformatics Commons, Chemicals and Drugs Commons, Computational Biology Commons, Genomics Commons
Notes
Cancer chemotherapy responses have been related to multiple pharmacogenetic biomarkers, often for the same drug. This study utilizes machine learning to derive multi-gene expression signatures that predict individual patient responses to specific tyrosine kinase inhibitors, including erlotinib, gefitinib, sorafenib, sunitinib, lapatinib and imatinib. Support Vector Machine learning was used to train mathematical models that distinguished sensitivity from resistance to these drugs using a novel systems biologybased approach. This began with expression of genes previously implicated in specific drug responses, then expanded to evaluate genes whose products were related through biochemical pathways and interactions. Optimal pathway-extended support vector machines predicted responses in patients at accuracies of 70% (imatinib), 71% (lapatinib), 83% (sunitinib), 83% (erlotinib), 88% (sorafenib) and 91% (gefitinib). These best performing pathway-extended models demonstrated improved balance predicting both sensitive and resistant patient categories, with many of these genes having a known role in cancer etiology. Ensemble machine learning-based averaging of multiple pathway-extended models derived for an individual drug increased accuracy to >70% for erlotinib, gefitinib, lapatinib, and sorafenib. Through incorporation of novel cancer biomarkers, machine learning-based pathway-extended signatures display strong efficacy predicting both sensitive and resistant patient responses to chemotherapy.