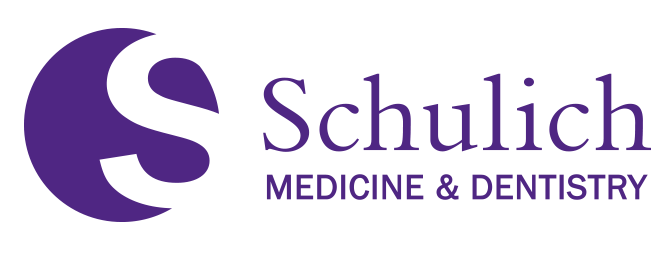
Medical Imaging Publications
Title
Contrast agent-free synthesis and segmentation of ischemic heart disease images using progressive sequential causal GANs.
Document Type
Article
Publication Date
5-1-2020
Journal
Medical image analysis
Volume
62
First Page
101668
Last Page
101668
URL with Digital Object Identifier
https://doi.org/10.1016/j.media.2020.101668
Abstract
The elimination of gadolinium contrast agent (CA) injections and manual segmentation are crucial for ischemic heart disease (IHD) diagnosis and treatment. In the clinic, CA-based late gadolinium enhancement (LGE) imaging and manual segmentation remain subject to concerns about potential toxicity, interobserver variability, and ineffectiveness. In this study, progressive sequential causal GANs (PSCGAN) are proposed. This is the first one-stop CA-free IHD technology that can simultaneously synthesize an LGE-equivalent image and segment diagnosis-related tissues (i.e., scars, healthy myocardium, blood pools, and other pixels) from cine MR images. To this end, the PSCGAN offer three unique properties: 1) a progressive framework that cascades three phases (i.e., priori generation, conditional synthesis, and enhanced segmentation) for divide-and-conquer training synthesis and segmentation of images. Importantly, this framework leverages the output of the previous phase as a priori condition to input the next phase and guides its training for enhancing performance, 2) a sequential causal learning network (SCLN) that creates a multi-scale, two-stream pathway and a multi-attention weighing unit to extract spatial and temporal dependencies from cine MR images and effectively select task-specific dependence. It also integrates the GAN architecture to leverage adversarial training to further facilitate the learning of interest dependencies of the latent space of cine MR images in all phases; and 3) two specifically designed self-learning loss terms: a synthetic regularization loss term leverages the spare regularization to avoid noise during synthesis, and a segmentation auxiliary loss term leverages the number of pixels for each tissue to compensate for discrimination during segmentation. Thus, the PSCGAN gain unprecedented performance while stably training in both synthesis and segmentation. By training and testing a total of 280 clinical subjects, our PSCGAN yield a synthetic normalization root-mean-squared-error of 0.14 and an overall segmentation accuracy of 97.17%. It also produces a 0.96 correlation coefficient for the scar ratio in a real diagnostic metric evaluation. These results proved that our method is able to offer significant assistance in the standardized assessment of cardiac disease.
Notes
This article is paywalled