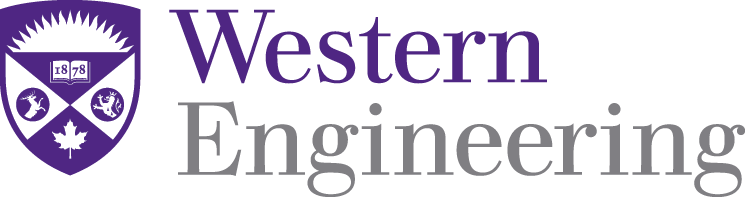
Electrical and Computer Engineering Publications
Document Type
Conference Proceeding
Publication Date
12-2020
Abstract
Nowadays, electricity demand forecasting is critical for electric utility companies. Accurate residential load forecasting plays an essential role as an individual component for integrated areas such as neighborhood load consumption. Short-term load forecasting can help electric utility companies reduce waste because electric power is expensive to store. This paper proposes a novel method to evolve deep neural networks for time series forecasting applied to residential load forecasting. The approach centres its efforts on the neural network architecture during the evolution. Then, the model weights are adjusted using an evolutionary optimization technique to tune the model performance automatically. Experimental results on a large dataset containing hourly load consumption of a residence in London, Ontario shows that the performance of unadjusted weights architecture is comparable to other state-of-the-art approaches. Furthermore, when the architecture weights are adjusted the model accuracy surpassed the state-of-the-art method called LSTM one shot by 3.0%.