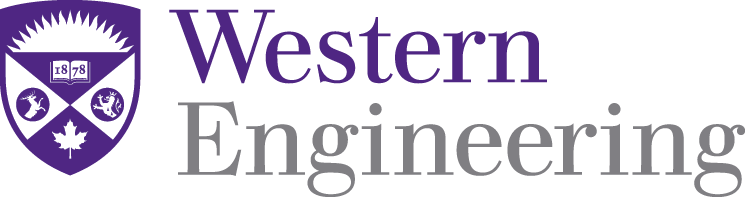
Electrical and Computer Engineering Publications
Document Type
Conference Proceeding
Publication Date
12-2020
Abstract
Electricity consumption is accelerating due to economic and population growth. Hence, energy consumption prediction is becoming vital for overall consumption management and infrastructure planning. Recent advances in smart electric meter technology are making high-resolution energy consumption data available. However, many parameters influencing energy consumption are not typically monitored for residential buildings. Therefore, this study’s main objective is to develop a data-driven energy consumption forecasting model (next-hour consumption) for residential houses solely based on analyzing electricity consumption data. This research proposes a deep neural network architecture that combines stationary wavelet transform features and convolutional neural networks. The proposed approach utilizes automatically extracted features from smart-meter readings by applying wavelet decomposition, convolution, and pooling operations. This study’s findings have demonstrated the advantage of integrating wavelet features with convolutional neural networks to improve forecasting accuracy while automating feature extraction.