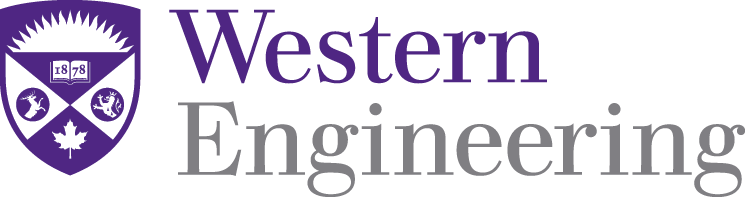
Electrical and Computer Engineering Publications
Document Type
Conference Proceeding
Publication Date
2019
Journal
Proc. of the IEEE Canadian Conference on Electrical and Computer Engineering
Abstract
Sensors, wearables, mobile and other Internet of Thing (IoT) devices are becoming increasingly integrated in all aspects of our lives. They are capable of collecting massive quantities of data that are typically transmitted to the cloud for processing. However, this results in increased network traffic and latencies. Edge computing has a potential to remedy these challenges by moving computation physically closer to the network edge where data are generated. However, edge computing does not have sufficient resources for complex data analytics tasks. Consequently, this paper investigates merging cloud and edge computing for IoT data analytics and presents a deep learning-based approach for data reduction on the edge with the machine learning on the cloud. The encoder part of the autoencoder is located on the edge to reduce data dimensions. Reduced data are sent to the cloud where there are used directly for machine learning or expanded to original features using the decoder part of the autoencoder. The proposed approach has been evaluated on the human activity recognition tasks. Results show that 50% data reduction did not have a significant impact on the classification accuracy and 77% reduction only caused 1% change.
Included in
Artificial Intelligence and Robotics Commons, Computer Engineering Commons, Electrical and Computer Engineering Commons, Software Engineering Commons
Notes
© 2019 IEEE. Personal use of this material is permitted. Permission from IEEE must be obtained for all other uses, in any current or future media, including reprinting/republishing this material for advertising or promotional purposes, creating new collective works, for resale or redistribution to servers or lists, or reuse of any copyrighted component of this work in other works